With unsupervised learning, no answer is specified. The data that is transferred therefore does not have to be logically structured.
The aim of this approach is to group similar elements. This is known as clustering. There are also numerous applications for this approach, such as presenting news articles on the same topic or introducing people with similar interests to each other in social networks. The criteria for clustering do not have to be known, but are learned. The interpretation must subsequently be carried out using human expert knowledge. In our example from printed circuit board production (for an introduction to the example, see the article on supervised learning), different errors can be assigned to different production parameters, as the figure illustrates. Here too, the number of parameters is not limited by the method.
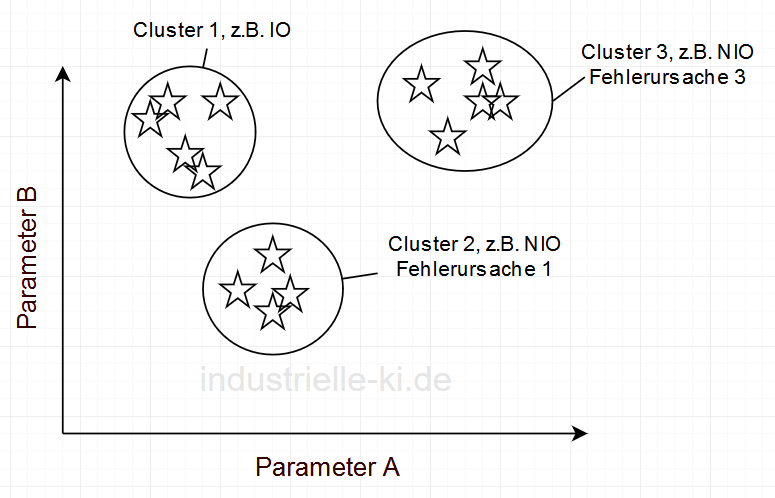